🤿 DeepLabv3+#
[Example] · [Architecture] · [Training Hyperparameters] · [Attribution] · [API Reference]
DeepLabv3+ is an architecture designed for semantic segmenation i.e. per-pixel classification. DeepLabv3+ takes in a feature map from a backbone architecture (e.g. ResNet-101), then outputs classifications for each pixel in the input image. Our implementation is a simple wrapper around torchvision’s ResNet for the backbone and mmsegmentation’s DeepLabv3+ for the head.
Example#
from composer.models import composer_deeplabv3
model = composer_deeplabv3(num_classes=150,
backbone_arch="resnet101",
backbone_weights="IMAGENET1K_V2",
sync_bn=False
)
Architecture#
Based on Encoder-Decoder with Atrous Separable Convolution for Semantic Image Segmentation
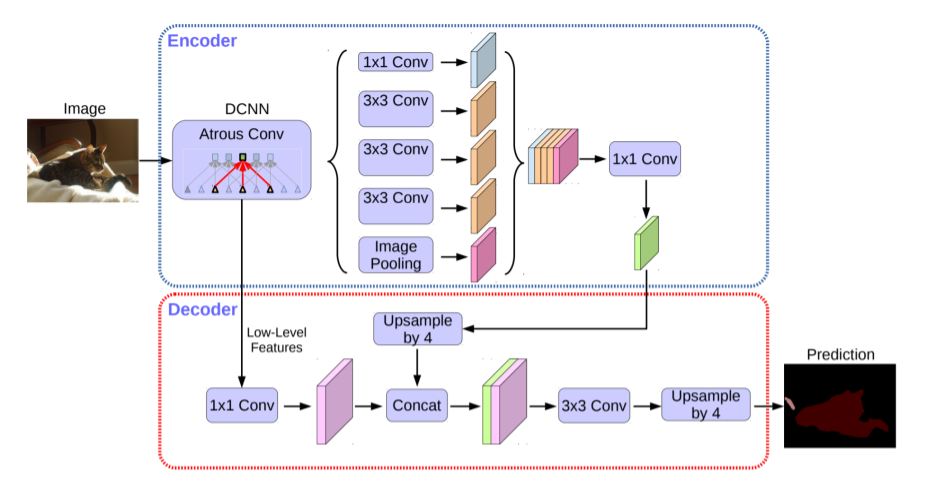
Backbone network: converts the input image into a feature map.
Usually ResNet-101 with the strided convolutions converted to dilations convolutions in stage 3 and 4.
The 3x3 convolutions in stage 3 and 4 have dilation sizes of 2 and 4, respectively, to compensate for the decreased receptive field.
The average pooling and classification layer are ignored.
Spatial Pyramid Pooling: extracts multi-resolution features from the stage 4 backbone feature map.
The backbone feature map is processed with four parallel convolution layers with dilations {1, 12, 24, 36} and kernel sizes {1x1, 3x3, 3x3, 3x3}.
In parallel to the convolutions, global average pool the backbone feature map, then bilinearly upsample to be the same spatial dimension as the feature map.
Concatenate the outputs from the convolutions and global average pool, then process with a 1x1 convolution.
The 3x3 convolutions are implemented as depth-wise convolutions to reduce memory and computation cost.
Decoder: converts the output of spatial pyramid pooling (SPP) to class predictions of the same spatial dimension as the input image.
SPP output is bilinearly upsampled to be the same spatial dimension as the output from the first stage in the backbone network.
A 1x1 convolution is applied to the first stage activations, then this is concatenated with the upsampled SPP output.
The concatenation is processed by a 3x3 convolution with dropout followed by a classification layer.
The predictions are bilinearly upsampled to be the same resolution as the input image.
Training Hyperparameters#
We tested two sets of hyperparameters for DeepLabv3+ trained on the ADE20k dataset.
Typical ADE20k Model Hyperparameters#
Model: deeplabv3:
Initializers: kaiming_normal, bn_ones
Number of classes: 150
Backbone weights: IMAGENET1K_V1
Sync BatchNorm
Optimizer: SGD
Learning rate: 0.01
Momentum: 0.9
Weight decay: 5.0e-4
Dampening: 0
Nsterov: false
LR schedulers:
Polynomial:
Alpha_f: 0.01
Power: 0.9
Number of epochs: 127
Batch size: 16
Precision: amp
Model |
mIoU |
Time-to-Train on 8xA100 |
---|---|---|
ResNet101-DeepLabv3+ |
44.17 +/- 0.17 |
6.385 hr |
Composer ADE20k Model Hyperparameters#
Model: deeplabv3:
Initializers: kaiming_normal, bn_ones
Number of classes: 150
Backbone Architecture: resnet101
Sync BatchNorm
Backbone weights: IMAGENET1K_V2
Optimizer: Decoupled SGDW
Learning rate: 0.01
Momentum: 0.9
Weight decay: 2.0e-5
Dampening: 0
Nesterov: false
LR schedulers:
Cosine decay, t_max: 1dur
Number of epochs: 128
Batch size: 32
Precision: amp
Model |
mIoU |
Time-to-Train on 8xA100 |
---|---|---|
ResNet101-DeepLabv3+ |
45.764 +/- 0.29 |
4.67 hr |
Improvements:
New PyTorch pretrained weights
Cosine decay
Decoupled Weight Decay
Increase batch size to 32
Decrease weight decay to 2e-5
Attribution#
Encoder-Decoder with Atrous Separable Convolution for Semantic Image Segmentation by Liang-Chieh Chen, Yukun Zhu, George Papandreou, Florian Schroff, Hartwig Adam
OpenMMLab Semantic Segmentation Toolbox and Benchmark
How to Train State-Of-The-Art Models Using TorchVision’s Latest Primitives by Vasilis Vryniotis
API Reference#
- class composer.models.deeplabv3.composer_deeplabv3(num_classes, backbone_arch='resnet101', backbone_weights=None, sync_bn=True, use_plus=True, ignore_index=- 1, cross_entropy_weight=1.0, dice_weight=0.0, initializers=())[source]
- Helper function to create a
ComposerClassifier
with a DeepLabv3(+) model. Logs Mean Intersection over Union (MIoU) and Cross Entropy during training and validation.
- From Rethinking Atrous Convolution for Semantic Image Segmentation
(Chen et al, 2017).
- Parameters
num_classes (int) – Number of classes in the segmentation task.
backbone_arch (str, optional) – The architecture to use for the backbone. Must be either [
'resnet50'
,'resnet101'
]. Default:'resnet101'
.backbone_weights (str, optional) – If specified, the PyTorch pre-trained weights to load for the backbone. Currently, only [‘IMAGENET1K_V1’, ‘IMAGENET1K_V2’] are supported. Default:
None
.sync_bn (bool, optional) – If
True
, replace all BatchNorm layers with SyncBatchNorm layers. Default:True
.use_plus (bool, optional) – If
True
, use DeepLabv3+ head instead of DeepLabv3. Default:True
.ignore_index (int) – Class label to ignore when calculating the loss and other metrics. Default:
-1
.cross_entropy_weight (float) – Weight to scale the cross entropy loss. Default:
1.0
.dice_weight (float) – Weight to scale the dice loss. Default:
0.0
.initializers (List[Initializer], optional) – Initializers for the model.
[]
for no initialization. Default:[]
.
- Returns
ComposerModel – instance of
ComposerClassifier
with a DeepLabv3(+) model.
Example:
from composer.models import composer_deeplabv3 model = composer_deeplabv3(num_classes=150, backbone_arch='resnet101', backbone_weights=None)
- Helper function to create a